Publicación: Modelo de Machine Learning para la predecir la destinación de un área cultivable
dc.contributor.advisor | Méndez Mejía, Santiago | |
dc.contributor.author | Calle Botero, Pablo Jose | |
dc.date.accessioned | 2024-06-25T14:25:55Z | |
dc.date.available | 2024-06-25T14:25:55Z | |
dc.date.issued | 2024 | |
dc.description | 41 páginas | |
dc.description.abstract | RESUMEN: los suelos productivos son un recurso finito el cual cada vez más es necesario darle un manejo adecuado además de ser optimizado al máximo para lograr la seguridad alimentaria de la población. El Banano de exportación, uno de los productos estrella de Colombia, ha visto el área que se le dedica para ser cultivado crecer en los últimos años pasando de 44,953 hectáreas en 2007 a unas 49,307 hectáreas en 2017. Con un crecimiento significativo del área cultivada se esperaría que la producción experimentará un crecimiento similar, pero ese no ha sido el caso. Año tras año el rendimiento lo cual es el número de toneladas cosechadas por hectárea ha disminuido. (Ministerio de Agricultura y Desarrollo Rural, 2020)Si un incremento en el área cultivada no representa un incremento en la producción puede ser por que el área nueva que se está destinando no es la adecuada y sus características están afectando el cultivo. La presencia natural de diferentes micronutrientes que se encuentran en el suelo ya sea por naturaleza o el uso de fertilizantes tienen diferentes funciones que son vitales para el desarrollo y vida de las plantas. La presencia del Cobre, Manganeso, Zinc y Boro son fundamentales para el crecimiento de las plantas. (Kyrkby & Römheld, 2007) Otro elemento fundamental y esencial para todo ser vivo incluidas las plantas es el Nitrógeno ya que este hace parte de los aminoácidos que componen las proteínas requeridas por todos los organismos. (Félix Herrán, Sañudo Torres, Rojo Martínez, Martínez Ruiz, & Olalde Portugal, 2008)Para determinar la presencia de estos elementos y muchos otros es necesario realizar estudios de suelo los cuales requieren de la toma de una muestra de un punto de interés que posteriormente es llevada a un laboratorio. Este proceso es altamente efectivo, pero requiere de mucho tiempo. Estos estudios se hacen para determinar la viabilidad del cultivo. Dicha determinación es vital para aprovechar al máximo el área disponible evitando desperdicios. Una vez se tiene esta información es crucial hacer la selección del producto a destinar la tierra pues no todo producto agrícola es apto para todo tipo de suelo o ubicación. Si se tiene la información del suelo, ubicación una herramienta que permita tomar la decisión de destinación de manera rápida y ágil sería de gran ayuda para los agricultores. ¿Será posible que el desarrollo de un prototipo de una herramienta la cual permita realizar estimaciones precisas de la destinación un área cultívale por medio del uso de machine learning y una base de datos con la composición de los suelos, su ubicación geográfica y su destinación agrícola? En este trabajo con el fin de predecir la destinación agrícola de un cultivo se creo un prototipo de modelo de machine learining con un conjunto de datos de diferentes zonas de Colombia. Este conjunto de datos de preparo y luego con base a este se probaron 5 modelos diferentes para tener una muestra diferente. Se concluye que se puede predecir con exactitud la destinación agrícola de un cultivo pero que se es necesario de más herramientas, pero modelos como este ayudan a la tecnificación del agro. | spa |
dc.description.abstract | ABSTRACT: Productive soils are a finite resource that increasingly requires proper management and optimization to achieve food security for the population. Export bananas, one of Colombia's star products, have seen the area dedicated to their cultivation grow in recent years from 44,953 hectares in 2007 to 49,307 hectares in 2017. With a significant increase in the cultivated area, one would expect production to experience similar growth, but this has not been the case. Year after year, the yield, which is the number of tons harvested per hectare, has decreased. (Ministry of Agriculture and Rural Development, 2020). If an increase in the cultivated area does not represent an increase in production, it may be because the new area that is being allocated is not suitable and its characteristics are affecting the crop. The natural presence of different micronutrients found in the soil, either naturally or through the use of fertilizers, have distinct functions that are vital for the development and life of plants. The presence of Copper, Manganese, Zinc, and Boron are essential for plant growth. (Kyrkby & Römheld, 2007). Another fundamental and essential element for all living beings, including plants, is Nitrogen, since it is part of the amino acids that make up the proteins required by all organisms. (Félix Herrán, Sañudo Torres, Rojo Martínez, Martínez Ruiz, & Olalde Portugal, 2008). To determine the presence of these elements and many others, it is necessary to carry out soil studies, which require taking a sample from a point of interest that is later taken to a laboratory. This process is highly effective, but it requires a lot of time. These studies are done to determine the viability of the crop. Such a determination is vital to make the most of the available area, avoiding waste. Once this information is available, it is crucial to select the product to allocate the land to, as not all agricultural products are suitable for all types of soil or location. If you have soil information and location, a tool that allows you to make the allocation decision quickly and efficiently would be of significant help to farmers. Would it be possible to develop a prototype of a tool that allows for accurate estimates of the allocation of a cultivation area through the use of machine learning and a database with the composition of the soils, their geographical location, and their agricultural use? In this work, with the aim of predicting the agricultural destination of a crop, a prototype of a machine learning model was created with a dataset from different areas of Colombia. This dataset was prepared and then based on this; 5 different models were tested to have a different sample. It is concluded that it is possible to accurately predict the agricultural destination of a crop, but that more tools are needed, but models like this help to modernize agriculture. | eng |
dc.description.degreelevel | Pregrado | |
dc.description.degreename | Ingeniero(a) de Sistemas y Computación | |
dc.format.mimetype | application/pdf | |
dc.identifier.uri | https://repository.eia.edu.co/handle/11190/6628 | |
dc.language.iso | spa | |
dc.publisher | Universidad EIA | |
dc.publisher.faculty | Escuela de Ingeniería y Ciencias Básicas | |
dc.publisher.place | Envigado (Antioquia, Colombia) | |
dc.publisher.program | Ingeniería de Sistemas y Computación | |
dc.rights | Derechos Reservados - Universidad EIA, 2024 | |
dc.rights.accessrights | info:eu-repo/semantics/openAccess | |
dc.rights.coar | http://purl.org/coar/access_right/c_abf2 | |
dc.rights.license | Atribución-NoComercial-SinDerivadas 4.0 Internacional (CC BY-NC-ND 4.0) | |
dc.subject.proposal | Machine Learning | eng |
dc.subject.proposal | Agricultura | spa |
dc.subject.proposal | Seguridad Alimenticia | spa |
dc.subject.proposal | Agriculture | eng |
dc.subject.proposal | Food Safety | eng |
dc.title | Modelo de Machine Learning para la predecir la destinación de un área cultivable | spa |
dc.type | Trabajo de grado - Pregrado | |
dc.type.coar | http://purl.org/coar/resource_type/c_7a1f | |
dc.type.coarversion | http://purl.org/coar/version/c_970fb48d4fbd8a85 | |
dc.type.content | Text | |
dc.type.driver | info:eu-repo/semantics/bachelorThesis | |
dc.type.redcol | http://purl.org/redcol/resource_type/TP | |
dc.type.version | info:eu-repo/semantics/publishedVersion | |
dspace.entity.type | Publication |
Archivos
Bloque original
1 - 1 de 1
Cargando...
- Nombre:
- PabloJoseCalleBotero_2024_ModeloMachineLearning
- Tamaño:
- 1.18 MB
- Formato:
- Adobe Portable Document Format
- Descripción:
- Trabajo de grado
Bloque de licencias
1 - 1 de 1
Cargando...
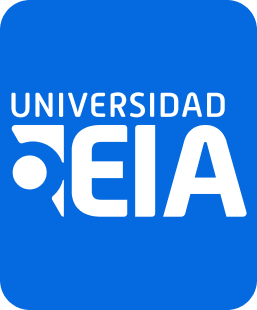
- Nombre:
- license.txt
- Tamaño:
- 2.49 KB
- Formato:
- Item-specific license agreed upon to submission
- Descripción: